What is Data Science Day?
The Data Science Day is organised and run every year by the Data Science Institute at Columbia University in New York City. The Data Science Institute trains the next generation of data scientists and develops technology to serve society. With more than 350 affiliated faculty members working in a range of disciplines, the Institute fosters collaboration in advancing techniques to gather and interpret data and addresses serious problems facing society. The Institute also works with industry to bring promising research ideas to market.
Usually, the event held by the Data Science Institute is an in-person event at its campus – however, due to the ongoing pandemic, there was no choice but to present it virtually. This presented the opportunity for remote visitors to join.
One such visitor was me, Andrew Bolt. Though based in the UK, and five hours ahead of New York time, I wanted to join due to the interest I had in the subject matter. I did so, given that I could this year.
The day itself was entitled “Ethics and Privacy: Terms of Usage”. From here, one may be tempted to skip to the end and click ‘Accept’, as many do for real terms and conditions. Yet, the conference covered a variety of topics, from disciplines as diverse as neurotechnology, GDPR and public health.
I was very fortunate to be able to attend Data Science Day 2020 and I wanted to share what happened and what I learned.
Format
Data Science Day 2020 started with an introduction from Jeanette Wing, Avanessians Director of the Data Science Institute at Columbia University and creator of the FATES model of data ethics.
Afterwards, four professors from the university discussed their work and insights in ‘Lightning Talks’, short presentations that covered their respective topics at a high level and in terms that were easy to understand and conceptualise.
However, the highlight of Data Science Day was the keynote from Eric Schmidt, co-founder of Google who transformed it from a small Silicon Valley start-up to one of the largest and most iconic companies on the planet.
Finally, Wing fielded questions to Schmidt on his experience in technology and the future of the industry as a whole.
The whole live stream, including the keynote address by Eric Schmidt, can be found here.
Lightning Talks
As a general theme, many of the talks delved into the intersection of human rights and technology. That is, the ethics involved in collecting data from various subjects, and respecting their importance concerning their provenance. A common thread that ran through each was the Belmont Report of 1979, with respect to the data rights of research subjects
The speakers of the talks were as follows:
The NeuroRights Initiative: Human Rights Guidelines for Neurotechnology and AI in a Post-COVID World
To understand what this technology is, think of Elon Musk’s recently released Neuralink. At its core, neurotechnology is concerned with collecting neuronal data using a brain-computer interface (BCI). The data are processed to measure neuronal activity, which can be used to spot neurological diseases.
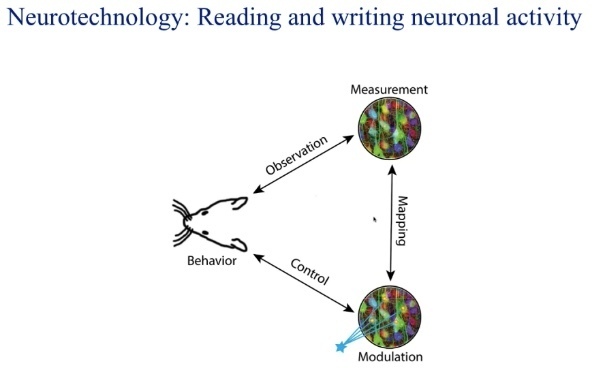
However, BCIs work both ways: they can be used to manipulate neuron activity. This does beg the question of the inception of ideas and manipulation. If our minds can be read to and written from like a hard disk, what does this say about our humanity?
Professor Yuste seeks to answer this question by ensuring a set of rights are enshrined into the Universal Declaration of Human Rights. These are the NeuroRights:
- The RIght to Personal Identity
- The Right to Free-Will
- The Right to Mental Privacy
- The Right to Equal Access to Mental Augmentation
- The Right to Protection from Algorithmic Bias
These are built upon research from the US Brain Initiative, a $6 billion US research programme into neurotechnology, collaboration from the Morningside Alliance Group at Columbia and the global BRAIN initiative. Over 15 years of research later, neurotechnology has advanced from being tested from worms to flies to mice and then to humans, in much the same way that the Human Genome by which it was inspired did.
Whilst the technology is more mature, the legislation is still being developed. That said, work with the Chilean government is being completed to add NeuroRights to its constitution.
On the 19th November, the symposium ‘Brain-Computer Interfaces: Innovation, Security and Society’ will be hosted online. You can register here.
The Effect of Privacy Regulation on the Data Industry: Empirical Evidence from GDPR
Whilst many of the talks have a focus on the United States, Professor Che’s talk was focussed on the effect of the European Union General Data Protection Regulation. For those who do not know, this is a regulation (meaning there is no flexibility in how it is implemented from region to region) that determines how data must be stored, collected, processed and moved. In the United Kingdom, this is enshrined into law as the Data Protection Act 2018, superseding the Act of 1998.
The question is: has the Regulation had any effect since its introduction? To answer this, a study was taken of those who opted out of cookies from a travel intermediary site that was compliant with GDPR. Before it came into effect, it was found that more users were circumventing data collections by obfuscation, deleting cookies or by using private browsing. However, as GDPR came into effect, opting out has become easier as it is required by law: consequently, the number of those opting out of data collection increased sharply.
Whilst this addressed the tension that consumers felt (they believe that there are too many forms, many of which have an unknown destination), it led to a loss of customers for the data processing firms. However, the remaining users were shown to be more viable and predictable.
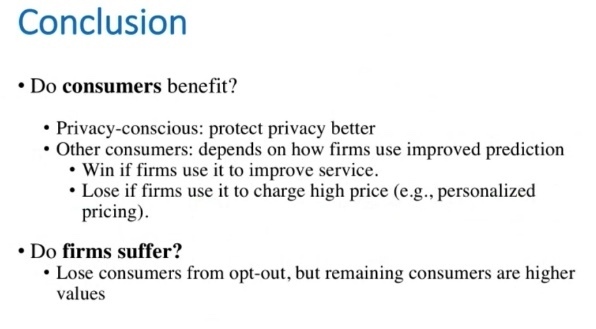
For more information on this, as well as the data itself, you can read ‘The Effect of Privacy Regulation on the Data Industry: Empirical Evidence from GDPR’ here.
Building a More Ethical Data Science: Lessons From Public Health
Professor Goldsmith gave a presentation with several caveats: he is not an ethicist but a biostatistician working in the field of public health. That said, he was able to provide a great deal of insight on the ethics of data collection.
The field of biostatistics is dominated by human-centred research: data are collected with the consent of human subjects, on the mutual understanding of its intended use. Therefore, each of the rows of a given data set represents attributes tied intimately to real people, such as heart rate or blood sugar levels. Outside of the fields of data science and public health, biostatistical data are still understood by the general public due to its visceral and the shared cognizance of its importance.
However, this is only viable when an ethical framework is applied. For example, in the Belmont Report, empathy is needed when researching with human subjects. Goldsmith’s lesson from this is to understand the significance of the data one is collecting, regardless of its origin. That is, data scientists are urged to understand the very real impact that the data have in the real world.
Don’t be evil…
Famously, Google’s motto was at one point “don’t be evil”. Professor Goldsmith outlined the importance of not just doing good with data, but also in not causing any form of harm. What this definition of harm is can, according to his findings, only be gauged from external oversight. This oversight can be used to weigh in upon issues of data ethics and fairness.
Post hoc ergo propter hoc?
If there is a correlation between two events, then the first must have caused the second? This is a fallacy that is often correctly pointed out from misleading data.
However, this does not always hold true. Sometimes, there is a causal relationship (correlation is not causation but it is not, not causation either). These causal relationships are more difficult to guess but are critical to getting right: one must understand where and from whom to draw inferences, how to measure causation and imperfections inherent in the sample data. The example that Goldsmith gave was the link between smoking and cancer, which is something now known to be causal.
To do this well, one must seriously interrogate the data. This may include gaining stakeholder input, such as the subjects themselves. This is to ensure that any biases about causality can be eliminated, especially if these biases impact on the real world.
Security and Privacy Guarantees in Machine Learning with Differential Privacy
Differential privacy is a statistical approach to protecting the personal data of a population. The individual records are deliberately obfuscated, whilst the statistical properties such as the arithmetic mean and variance are shared between data processing partners.
Whilst this may appear to be a panacea for obscuring personal data and also extracting value customer insight, individual purchase data can still be exposed. It is still advisable to exercise caution when using Differential Privacy, such as in the case that Professor Geambasu elucidated where the population of the data set is 1.
Moreover, a study by Dinur and Nissim in 2018 concluded that the 2010 US Census database was reconstructed, showing gender and ethnicity records for 52 million Americans. Ultimately, according to Geambasu, this was achieved by solving a large series of linear equations, possible with current supercomputing capabilities.
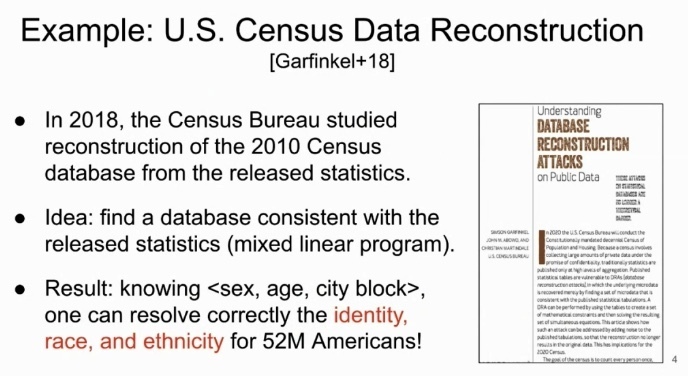
As Geambasu concluded, deep neural networks are also affected by data reconstruction when their model parameters are shared. In order to counteract this, data must be randomised so that no single point has any effect on the overall distribution.
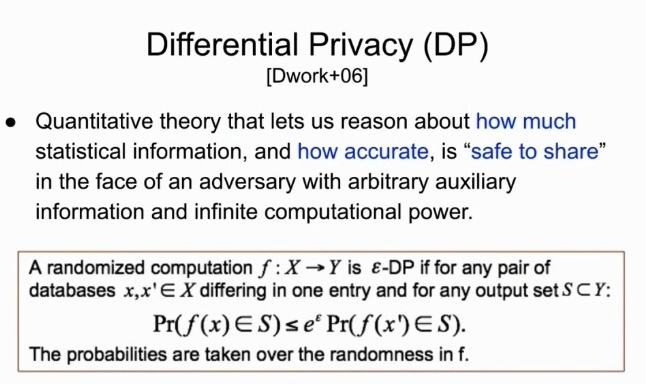
Practically, there is little progress in implementing Differential Privacy compared with the maturity of the algorithmic discipline. That said, the FDA adverse event database is now applying Differential Privacy urgently due to the sensitive nature of its data.
Keynote: Eric Schmidt
The final part of the Data Science Day 2020 was a keynote address from co-founder of Google and Schmidt Futures, which was recently granted a Blue Ribbon from New York’s Governor Cuomo to fight COVID-19.
Technology Idealism
Schmidt approached the keynote with optimism, though he remarked that he could get angry when the reality of the world collides with technological idealism. This was partly due to a naiveté that technologists have about the use cases of what they invent and bring into the world, and how they affect others. For example, the use of social media to stoke radicalism and eroding democracy.
In this regard, there are many inventions that are technologically mature but do not have the corresponding ethical framework. An example that Schmidt cites is facial recognition: whilst it is now possible to track the smallest facial attributes, there is still limited conversation on how they are being used, such as to track entire populations.
With the advent of open-source, inventors are free to showcase their technology for everyone in the world to use. However, this often goes without guidelines on who and how the source code is to be used. It is true that everyone all over the world can access technology hosted on services such as Git, but that also includes those who are antagonistic. Arguably, there needs to be a greater dialogue on what it means to be responsible as an innovator.
Re-addressing facial recognition, Schmidt commented on the biases in technology that stem from it, such as differences in recognition for ethnic minorities. As Cathy O’Neil once said, “algorithms are opinions embedded in code” – this implies that the conscious or unconscious biases of the programmers are written into how the code works. I would argue that a diverse base of programmers can be a start, but Schmidt also argues that technology should not be done in a vacuum so that conversations about biases can arise.
Moreover, the current drive to provide solutions to problems (at least from a technological standpoint) means that unforeseen problems may arise after the solution of a given problem. Schmidt argues that there needs to be dialogue to explore what these problems would be and how to solve them. From my experience, I have found that a problem-centred approach to software design helps: whilst more time is spent on defining the problem, the remaining build time is more useful as it directly solves the problem being posed.
Authoritarianism vs Democracy
Due to the rise in influence of data harvesting in commerce, technology and wider society, Schmidt raised the fight between democracy and authoritarianism in technology. As mentioned earlier, social media has had the unintended consequence of creating a more distrusting society, fractured into smaller and smaller social bubbles. The world is becoming increasingly decoupled, and the implications of this are bad for the future of the industry and for the planet.
Currently, there is an aphorism that “data is the new oil”. This is certainly true according to Schmidt who, with his breadth of experience and knowledge, predicts that companies that rely on it for their AI models that are increasingly becoming their entire business models.
How these are used is a question that must be begged: as opinions written in code, the AI models that we create differ from country to country. Schmidt himself cited the difference between China and the United States, with the former adopting a stack that is one or two generations ahead of the rest of the world, but for the purposes of constant surveillance for its citizens. I believe that one only has to examine the social credit system and the tracking of Uighur Muslims to see how China uses its AI stack.
One model in opposition to this is to grant the ownership of data to its subjects: Professor Yuste covered this concept earlier by stating that data from BCIs should be treated as any other human organ. However, Schmidt argues that countries need to imbue their values into how they use technology, raising the point that engineers and data scientists need their own version of American exceptionalism.
This raises the question of the difference between countries. As Schmidt elucidated, there is a difference between how countries believe in the data processing mores of the different sectors in society. This table shows this difference for the examples that Schmidt gave:
Country | Sector | Trust |
---|---|---|
UK | Government | Yes |
UK | Business | No |
USA | Government | No |
USA | Business | Yes |
Germany | Government | No |
Germany | Business | No |
From a British perspective, this insight is very interesting for the UK, given that we have the highest number of street cameras in the world.
Regulation
Data protection regulation governs how data is to be used, processed, transported and stored. According to the EU General Data Protection Regulation, this must be done in an adequate, timely and relevant manner.
Though this is a general tenet for many other data protection regulations, the European Union is distinct in that it was the first international body to adopt the Right to be Forgotten. This is the right for data subjects to have any information about themselves deleted from records, such as crime databases. However, the law is currently predicated on the notability of the data subject. Whilst this may not seem objectionable at first, one must then ask who determines what is notable? According to Schmidt, this is a function that a government should take on, rather than the companies holding the data themselves (such as Google).
Whilst regulation for future technologies, such as outlined in NeuroRights and other future initiatives, Schmidt argues the pragmatic view that current technology should take precedence in being legislated, as it is more effective to legislate what is actually in reality. Moreover, legislating for the future assumes a knowledge of what the future is like, including any ramifications and unintended consequences that simply cannot be known.
Conclusion
In conclusion, I really gained a lot of this event. I firmly believe that this type of academic discussion would be very insightful for anyone who uses technology at any level.
Thank you also to the Data Science Institute at Columbia for organising, our team looks forward to more events in the future.